Where Facebook AI research moves next
Five years is an awful lot of time in the tech industry. Darling startups find ways to crash and burn. Trends that seem unstoppable sputter-out. In the field of artificial intelligence, the past five years have been nothing short of transformative.
Facebook’s AI Research lab (FAIR) turns five years old this month, and just as the social media giant has left an indelible mark on the broader culture — for better or worse — the work coming out of FAIR has seen some major impact in the AI research community and entrenched itself in the way Facebook operates.
“You wouldn’t be able to run Facebook without deep learning,” Facebook Chief AI Scientist Yann LeCun tells TechCrunch. “It’s very, very deep in every aspect of the operation.”
Reflecting on the formation of his team, LeCun recalls his central task in initially creating the research group was “inventing what it meant to do research at Facebook.”
“Facebook didn’t have any research lab before FAIR, it was the first one, until then the company was very much focused on short-term engineering projects with six-month deadlines, if not less,” he says.
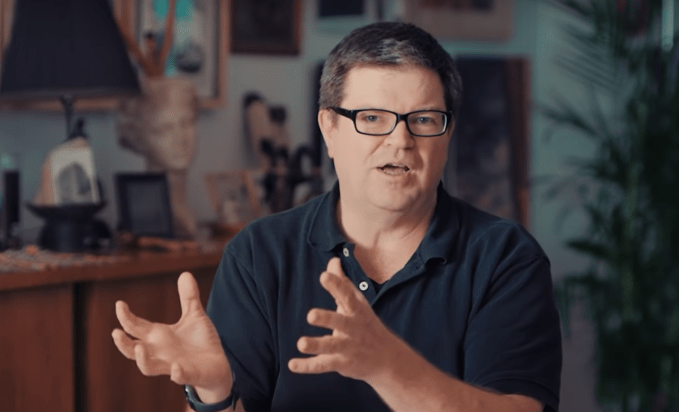
LeCun
Five years after its formation, FAIR’s influence permeates the company. The group has labs in Menlo Park, New York, Paris, Montreal, Tel Aviv, Seattle, Pittsburgh and London. They’ve partnered with academic institutions and published countless papers and studies, many of which the group has enumerated in this handy five-year anniversary timeline here.
“I said ‘No’ to creating a research lab for my first five years at Facebook,” CTO Mike Schroepfer wrote in a Facebook post. “In 2013, it became clear AI would be critical to the long-term future of Facebook. So we had to figure this out.”
The research group’s genesis came shortly after LeCun stopped by Mark Zuckerberg’s house for dinner. “I told [Zuckerberg] how research labs should be organized, particularly the idea of practicing open research.” LeCun said. “What I heard from him, I liked a lot, because he said openness is really in the DNA of the company.”
FAIR has the benefit of longer timelines that allow it to be more focused in maintaining its ethos. There is no “War Room” in the AI labs, and much of the group’s most substantial research ends up as published work that benefits the broader AI community. Nevertheless, in many ways, AI is very much an arms race for Silicon Valley tech companies. The separation between FAIR and Facebook’s Applied Machine Learning (AML) team, which focuses more on imminent product needs, gives the group a “huge, huge amount of leeway to really think about the long term,” LeCun says.
I chatted with LeCun about some of these long-term visions for the company, which evolved into him spitballing about what he’s working on now and where he’d like to see improvements. “First, there’s going to be considerable progress in things that we already have quite a good handle on…”
A big trend for LeCun seems to be FAIR doubling down on work that impacts how people can more seamlessly interact with data systems and get meaningful feedback.
“We’ve had this project that is a question-and-answer system that basically can answer any question if the information is somewhere in Wikipedia. It’s not yet able to answer really complicated questions that require extracting information from multiple Wikipedia articles and cross-referencing them,” LeCun says. “There’s probably some progress there that will make the next generation of virtual assistants and data systems considerably less frustrating to talk to.”
Some of the biggest strides in machine learning over the past five years have taken place in the vision space, where machines are able to parse out what’s happening in an image frame. LeCun predicts greater contextual understanding is on its way.
“You’re going to see systems that can not just recognize the main object in an image but basically will outline every object and give you a textual description of what’s happening in the image, kind of a different, more abstract understanding of what’s happening.”
FAIR has found itself tackling disparate and fundamental problems that have wide impact on how the rest of the company functions, but a lot of these points of progress sit deeper in the five-year timeline.
FAIR has already made some progress in unsupervised learning, and the company has published work on how they are utilizing some of these techniques to translate between languages for which they lack sufficient training data so that, in practical terms, users needing translations from something like Icelandic to Swahili aren’t left out in the cold.
As FAIR looks to its next five years, LeCun contends there are some much bigger challenges looming on the horizon that the AI community is just beginning to grapple with.
“Those are all relatively predictable improvements,” he says. “The big prize we are really after is this idea of self-supervised learning — getting machines to learn more like humans and animals and requiring that they have some sort of common sense.”